Keynote speakers
In this edition of CBIC, we have the pleasure of having the following top-notch researchers as keynote speakers:
Prof. Dhiya Al-Jumeily
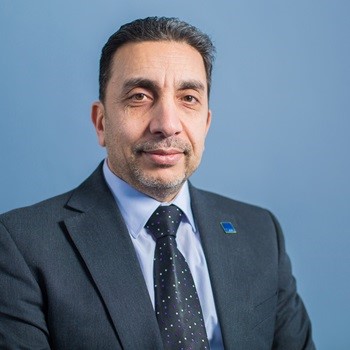
Professor of Software Engineering / Associate Dean, Faculty of Engineering and Technology, Liverpool John Moores University. web
Applications of Artificial Intelligence in Healthcare and Medicine
Applied Computing is the practice of embedding the realisation of Computer Science's latest technological advancements into industrial, business, and scientific intelligent solutions. Applied Computing stretches to a variety of fields, requiring an extensive knowledge of the specialised subject area and in many cases large teams of trained individuals to put into production. Applied Artificial Intelligence is considered as one of the major fields of Applied Computing. Artificial Intelligence has been introduced as an important tool in the implementation of Health, Business, Education, Entitlement, Tourism and more centred solutions as real world applications. The acceleration of technological change, especially the rise of mass computational availability in the late 20th Century, has led to the emergence of process and task frameworks that leverage and depend upon technological solutions. Subsequently, the use and acceptance of technology on a wide scale has opened up a new and expanding space of possibilities, allowing us to address many problems that were previously considered intractable, including the redefining the roles of many experts. Meanwhile, the long standing challenge of human healthcare has become a natural domain of interest for the incorporation of such advances in technology, since successful solutions in health translate into healthier populations and improve the quality of life of individuals. In general, the drive towards such technology rich applications, for the purpose of exploring new solution spaces, has been accompanied by an increasing rise in the need to effectively synthesis and manipulate information problems. The healthcare domain is no exception to this phenomenon.
The theme of Artificial Intelligence transcends computing. However, computing is perhaps a field at the forefront of exploring intelligence for the purpose of practical benefits to human society. It is arguable, though informative, to consider the dawn of computational intelligence as a consequence of the ideas of Alan Turing and the progression of computation from fixed immutable programs, manifested in hardware configurations, to entirely software based representations which provide the necessary potential and flexibility for self-modification and reflexivity as considered to be necessary conditions of intelligence. It is recognised that Turing was a key driving force in the paradigm shift from hardware driven designs in computing to the realisation that computation is in fact universal and can be represented by a machine capable of spanning the space of all possible computations, without the need for specialist hardware realisations for each and every class of task considered. The transcendence of computation from explicit hardware to universal machines parallels developments such as that of the wheel and the shift in thinking experienced during the industrial revolution. Such developments however point to another important consideration, that perhaps the uniquely human processes of thought and intelligence may not be exclusively locked into the biological substrate, and may instead be contained and cultivated independently using modern computational platforms as a suitable carrier. In this talk we explore the intersection of data science, big data, and the role of AI in healthcare/medicine, providing a background to problem domains, considering the progress so far, assessing the potential of such approaches, and exploring possible future directions.
The theme of Artificial Intelligence transcends computing. However, computing is perhaps a field at the forefront of exploring intelligence for the purpose of practical benefits to human society. It is arguable, though informative, to consider the dawn of computational intelligence as a consequence of the ideas of Alan Turing and the progression of computation from fixed immutable programs, manifested in hardware configurations, to entirely software based representations which provide the necessary potential and flexibility for self-modification and reflexivity as considered to be necessary conditions of intelligence. It is recognised that Turing was a key driving force in the paradigm shift from hardware driven designs in computing to the realisation that computation is in fact universal and can be represented by a machine capable of spanning the space of all possible computations, without the need for specialist hardware realisations for each and every class of task considered. The transcendence of computation from explicit hardware to universal machines parallels developments such as that of the wheel and the shift in thinking experienced during the industrial revolution. Such developments however point to another important consideration, that perhaps the uniquely human processes of thought and intelligence may not be exclusively locked into the biological substrate, and may instead be contained and cultivated independently using modern computational platforms as a suitable carrier. In this talk we explore the intersection of data science, big data, and the role of AI in healthcare/medicine, providing a background to problem domains, considering the progress so far, assessing the potential of such approaches, and exploring possible future directions.
Prof. Marc Schoenauer
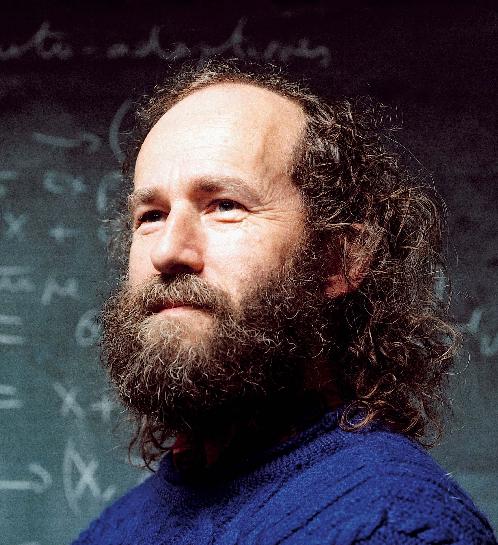
Directeur de Recherche, TAU Team, INRIA-Saclay/LRI/Université Paris-Saclay. web
Adaptation in Artificial Systems: lessons from Evolution Strategies applied to Deep Learning (slides)
Comparison to random behavior can help monitor and automatically control an optimization process, as demonstrated more than 20 years ago by the CMA-ES algorithm (Covariance Matrix Adaptation Evolution Strategy), today recognized as one of the best performing Black Box continuous optimizer. The first part of the talk will first survey CMA-ES adaptation mechanism, but will also provide evidence that the hyperparameters that control CMA-ES covariance matrix adaptation could in turn be tuned to the problem at hand, presenting EPM-CMA-ES, a Per Instance Algorithm Configuration for CMA-ES that won the GECCO Black-Box Optimization Competition last July.
The second part of the talk will introduce S-ALERA, yet another optimizer for Deep Learning: Based on a similar principle of comparison with a random walk, ALERA (Agnostic LEarning Rate Adaptation) gracefully increases or decreases the learning rate of Stochastic Gradient Descent. However, whereas allowing the learning rate to also increase can indeed speed-up the optimization, it makes the algorithm more prone to catastrophic events. A statistical test using Page-Hinkley change point detection is hence added, in order to detect such events, and then cool down the optimization process. The resulting algorithm (SALERA -- Safe ALERA) compares favorably to the state-of-the-art on standard benchmarks, while limiting the risks of dramatic explosions.
The second part of the talk will introduce S-ALERA, yet another optimizer for Deep Learning: Based on a similar principle of comparison with a random walk, ALERA (Agnostic LEarning Rate Adaptation) gracefully increases or decreases the learning rate of Stochastic Gradient Descent. However, whereas allowing the learning rate to also increase can indeed speed-up the optimization, it makes the algorithm more prone to catastrophic events. A statistical test using Page-Hinkley change point detection is hence added, in order to detect such events, and then cool down the optimization process. The resulting algorithm (SALERA -- Safe ALERA) compares favorably to the state-of-the-art on standard benchmarks, while limiting the risks of dramatic explosions.
Prof. José Manuel Molina
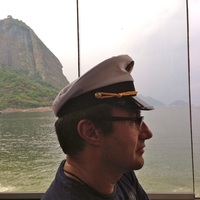
Full Professor, GIAA, Departamento de Informática, Universidad Carlos III de Madrid. web
Data Fusion and Computational Intelligence (slides)
Nowadays, Information Fusion systems addressing real-world problems must face a complex design process to allow the satisfaction of requirements for the selected application. In general, advanced IF systems are required to show robust, context-sensitive behavior, and efficient performance in real time. System designer must handle different sources of knowledge besides the specific sensor data to accomplish these objectives: information models and reasoning strategies to process data, and context knowledge about a priori relationships between entities and environment or constraints over the system output. To solve complex and real-world problems, computational intelligence techniques could be a good approach for solving these kind of problems. In this conference, we cover the application of hybrid systems in data fusion solutions with sensor data and high-level representations of situations, adaptation mechanisms or distributed collaborative techniques. Computational Intelligence methods can be useful in complex or specific designs, where features such as learning, reasoning, coordinated interaction, etc., are necessary to deal with dynamic situations whose characteristics cannot be precisely predicted during design time. The development of computation intelligence techniques brings new opportunities to improve classical sensor fusion systems. This is especially applicable in new domains requiring a high degree of customized sensor fusion, many demanding also from robust solutions to operate in a operational conditions, usually dealing with wrong data, unpredicted behaviors, constraints, etc. In these cases, a representation of domain knowledge and appropriate heuristics can be the only way to develop acceptable solutions, combining the use of classical fusion techniques with higher level criteria to drive the system behavior.